Comparison of CNV analysis methods: Array CGH vs NGS
- Insights | 22. 01. 06
Rare genetic disorders are caused by variants in major functional genes. Most are SNV or INDEL variants, but SVs, such as CNVs or chromosomal variants, can also be the cause.
Recently, a large-scale study has also been published showing that CNVs were identified in 11-12% more infants and children with neurodevelopmental disorders, mental retardation, and developmental disabilities than in the control group.2,3
What is the most suitable analysis method for CNV analysis? How might CNV analysis by whole exome sequencing be possible with short reads (150~300bp)? In this post, we will examine the characteristics and limitations of two common methods of CNV analysis.
array CGH (comparative genomic hybridization)
This is the method that has been the standard recommendation for CNV analysis.
First of all, microarray is a method that detects the sequence matching the probe by planting a probe that recognizes a specific sequence in each well. This is suitable when the scope of analysis is determined.
Array CGH is a method mainly used to detect quantitative abnormalities (deletion or duplication) of chromosomes. After attaching fluorescence materials Cy3 and Cy5 to the patient samples and control samples, respectively, the intensity of the fluorescence is compared to check for quantitative anomalies.
For example, if there is a deletion, the ratio of the control sample would be high and it would be red; if there is a duplication, the ratio of the patient sample would be high and it would be green. The resolution of aCGH is determined by the probe type, amount, and area mounted on the array.4
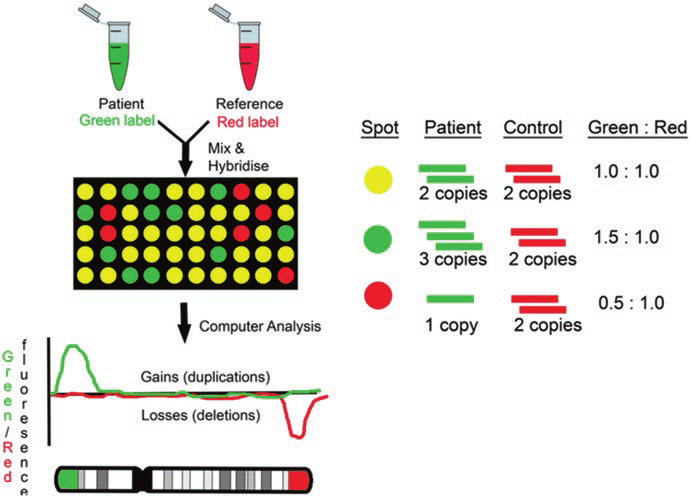
CNV Analysis Using Exome Data
The figure below shows the SV analysis method using NGS data, divided into 4 categories.5
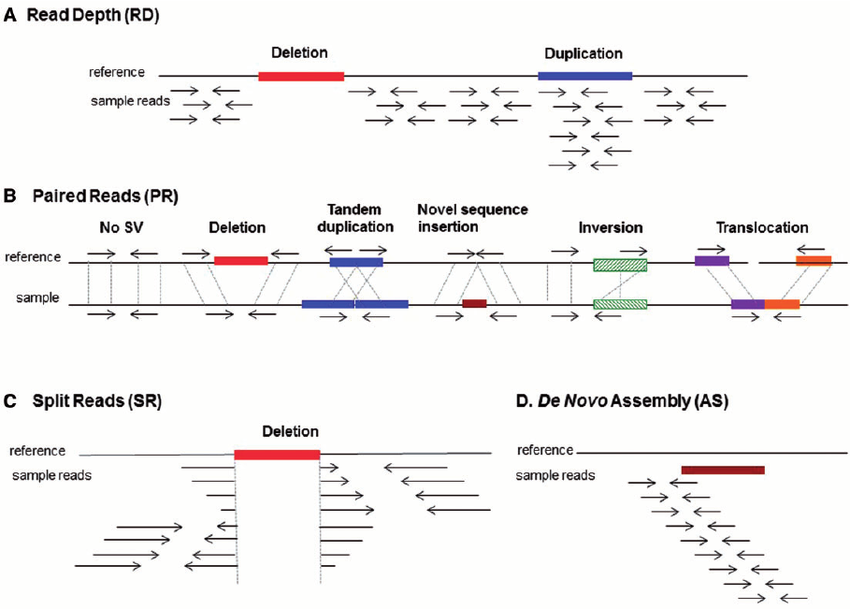
(A) Read depth: Relative comparison of depth between regions
(B) Paired Reads (PR): Unlike the target fragment size, when the distance between paired reads is far apart, too close, or there is a change in direction, etc.
(C) Split Reads: When one read is split and mapped to a reference
(D) De novo assembly: A sequence that is not in the reference is generated → insertion
Among them, CNV analysis based on whole exome sequencing uses (A) read depth. If the read depth of the area of analysis is sufficient and the read depth of a specific region decreases or increases rapidly, deletion and duplication may be suspected.
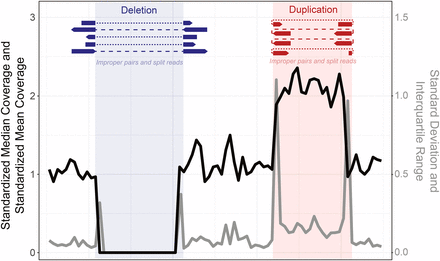
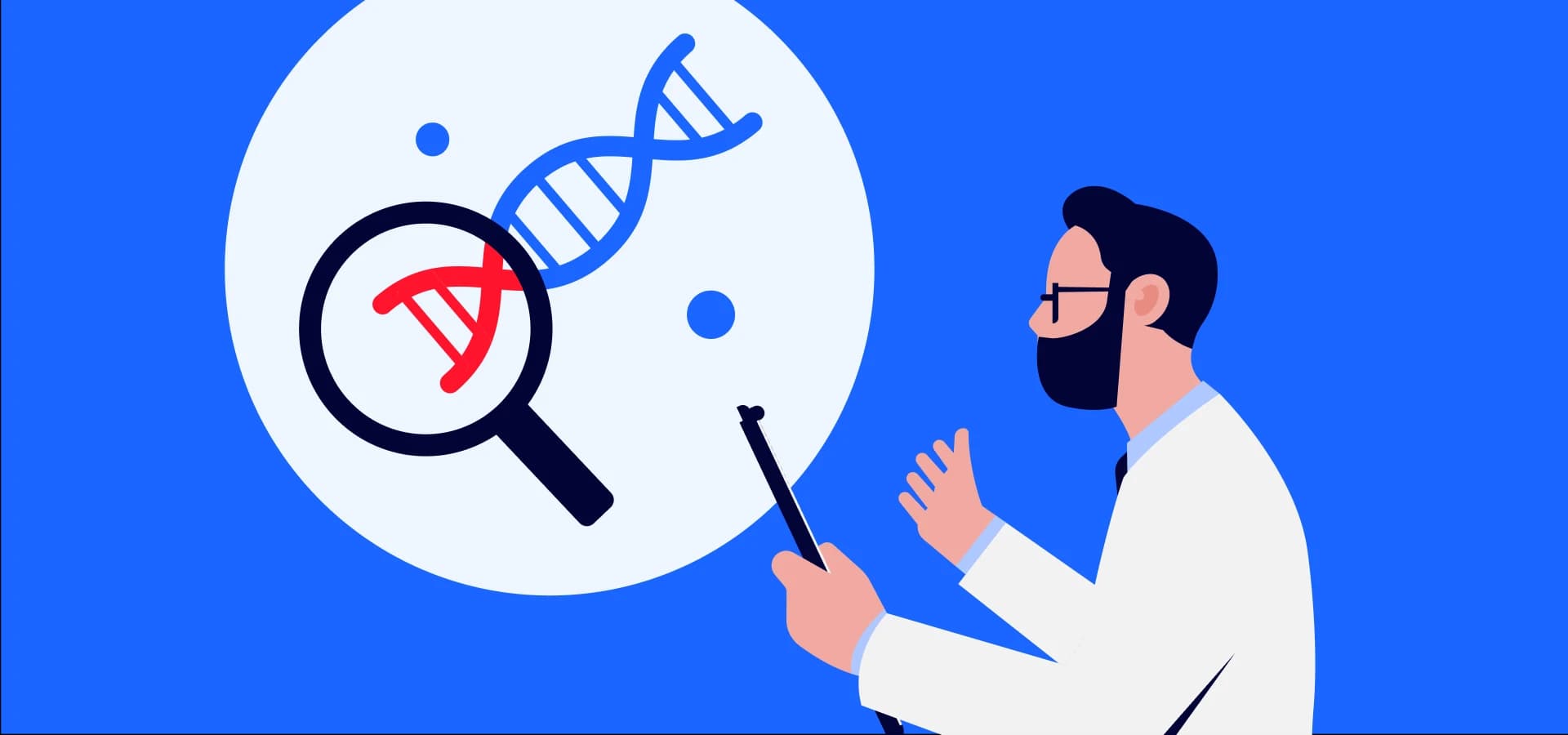
Comparison of aCGH and Clinical Exome sequencing
After dividing the 1412 patients with NDD symptoms who participated in the aCGH study into 3 major symptom groups (GDD/ASD/Other), a total of 245 patients who were not diagnosed through aCGH were selected from each group, and clinical exome sequencing was performed for them2. Results showed that 49 out of 245 people (20%) were diagnosed. There was a difference in the additional CES diagnosis rate depending on the symptoms (unlike the ASD group, the GDD group showed a significant improvement in diagnosis rate), and the more specific the symptoms were, the higher the diagnosis rate was. Though not all samples were analyzed both ways and thus there is a limitation for comparison, the diagnosis rate of the CES method was higher than that of aCGH (5.7% vs 20%). Since CES was performed with samples not diagnosed with aCGH, it can be predicted that the diagnostic rate by CES for all samples would be over 20%.
Why is there a difference?
- Difference in CGH resolution: In this experiment, 60 K aCGH (over 245 known genetic disorders and 980 gene regions known to be related to development) was used, but if the resolution is increased to 180 K, 400 K, or 1 M, the diagnosis rate may increase.
- Limitations of CES: Although the number of NDD genes reported by the Deciphering Developmental Disorders Consortium (2020) is around 2,000, CES contains only 1,400 gene regions, which is different from the regions covered by aCGH or WES, which may result in differences in diagnostic results.
- Lack of standardization of algorithms: Differences occur depending on the analysis lab → CNVs must be determined comprehensively by manually checking the coverage plot and normalized depth of the suspected area rather than relying on an automated tool.
Suggestion
Although the standard method for CNV analysis is still presented as MLPA (Multiple Ligation Dependent Probe Amplification) or aCGH, aCGH has the disadvantage of the difficulty in detecting exon CNV and MLPA the incapability of targeting multiple genes at once.6
As an example, a patient had congenital hearing defect, failure to thrive, hypotonia, and persistent abnormal liver function, and thus underwent array CGH elsewhere but could not find a causal variant. At 3billion, we performed whole exome sequencing on the patient’s sample to confirm SNV and Indel, but it was still difficult to make a diagnosis.
Afterwards, continuous reanalysis was carried out, and a 82.6kb deletion was suspected in the Xq28 region by the CNV analysis algorithm. The results were reported to the physician and a diagnosis could be made with these results.
Therefore, the optimal test method may differ depending on the type of disease suspected through the symptoms.
- aCGH is best for finding large gain and/or loss of DNA copies
- MLPA is suitable when there is a well-defined gene and for identifying an exon CNV (e.g. dup/del of PKD1/PKD2 which causes polycystic kidney disease)
- Through exome sequencing, SNVs and CNVs can be analyzed at the same time, simplifying the diagnostic process, or CNV of various sizes can be analyzed, which is particularly suitable for cases of large genetic heterogeneity or regions of genes with high homology, such as neurodegenerative or neurodevelopmental disorders. However, the focus is on read depth-based CNV gain/loss, so CNVs that cross over into non-coding regions cannot be analyzed.
- Through whole genome sequencing, even non-coding regions not covered by WES can be analyzed, so it is the most integrated analysis method that can check for mobile element insertion and translocation using paired read information.
As such, there is not one correct answer for diagnosis, so it is very important to understand the characteristics of different analysis methods accurately and to not give up on diagnosis with the results of just one test.
Recently, various research results have argued that the guidelines for CNV diagnosis should be changed to WES or WGS in order to incorporate new information and continuously confirm test results.
The direction of the arrow is changing. Experience diagnosis through a new direction.
References
- R Truty et.al., Prevalence and properties of intragenic copy-number variation in Mendelian disease genes. Genomic Medicine 21, 114 (2019)
- F Martinez-Granero et. al., Comparison of the diagnostic yield of aCGH and genome-wide sequencing across different neurodevelopmental disorders. npj Genomic Medicine 6, 25 (2021)
- B Royer-Bertrand et. al., CNV Detection from Exome Sequencing Data in Routine Diagnostics of Rare Genetic Disorders: Opportunities and Limitations. Genes 12, 1427 (2021)
- U. Qaisar et. al., Techniques of Chromosomal Studies. Chromosome Structure and Aberrations 14, 307 (2017)
- G Escaramís et. al., A decade of structural variants: description, history and methods to detect structural variation. Brief Funct Genomics 14, 305 (2015)
- ACMG Laboratory Quality Assurance Committee. American College of Medical Genetics and Genomics technical standards and guidelines: microarray analysis for chromosome abnormalities in neoplastic disorders. Genetics in Medicine 15, 484 (2013)
- https://www.biocompare.com/Editorial-Articles/363086-CNV-Analysis-Shifts-Focus-to-NGS-Sequences
Do you find this post helpful?
Click the button below to copy and share the link.
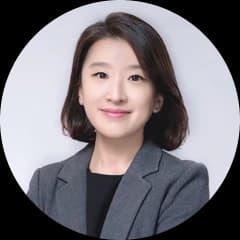
Sookjin Lee
Expert in integrating cutting-edge genomic healthcare technologies with market needs. With 15+ years of experience, driving impactful changes in global healthcare.