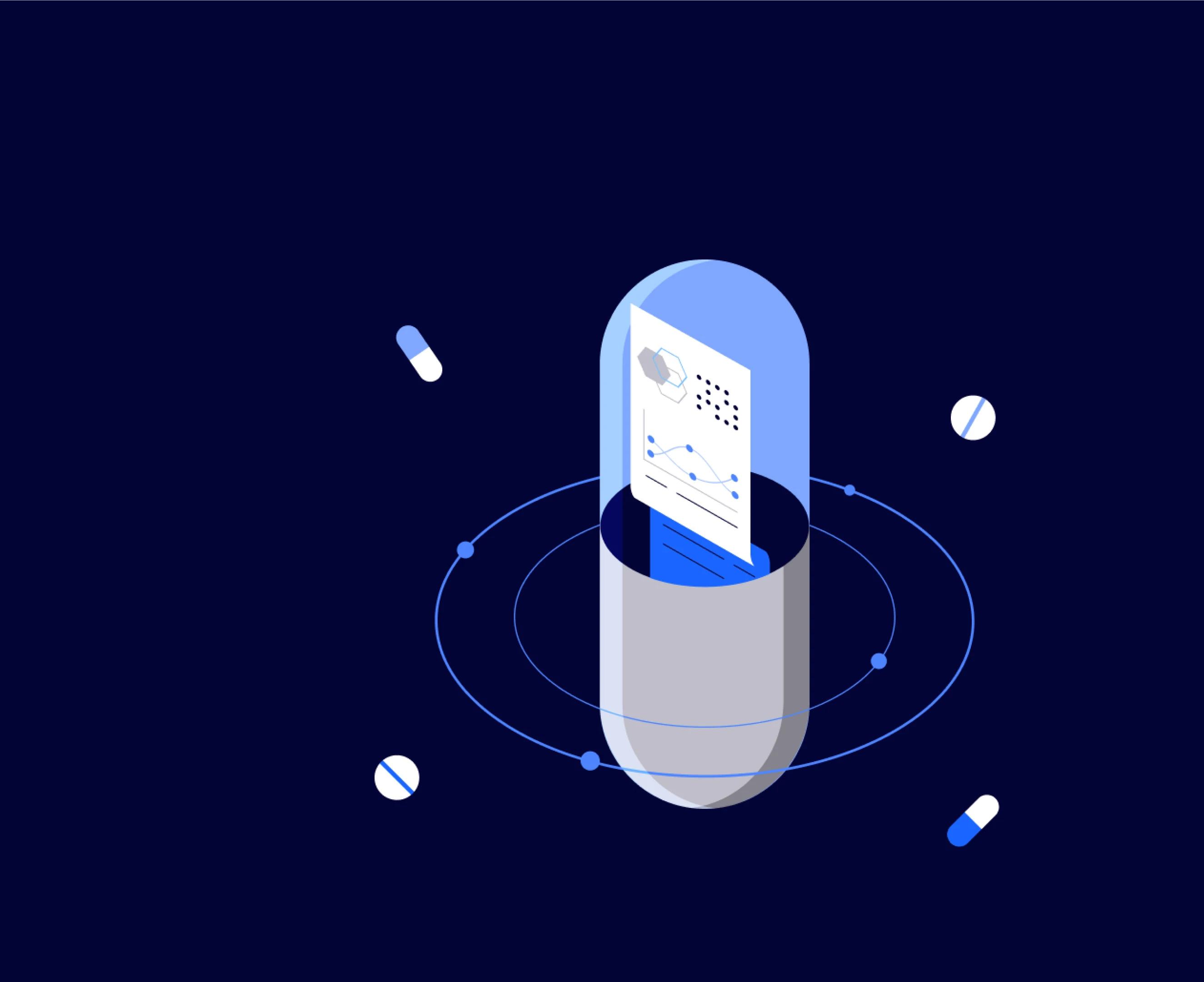
discovering new drug candidates
Limitations of existing deep learning drug discovery models
There have been attempts to create new compounds using deep learning methodologies.
However, there are limitations, such as finding a candidate with sufficient binding ability to the drug target, a candidate that can actually be produced in a lab, and a candidate that has enough flexibility to be optimized for many properties.
Application of AI systems to drug discovery
Utilizing patient genomic data to discover novel targets and pockets for small molecules so that we can treat the diseases known to be unDRUGGABLE TARGET.
Target Discovery
- Finding modifier gene for the disease using WES/WGS patient data
- Finding unknown GoF mechanism diseases which could be promising targets for SM
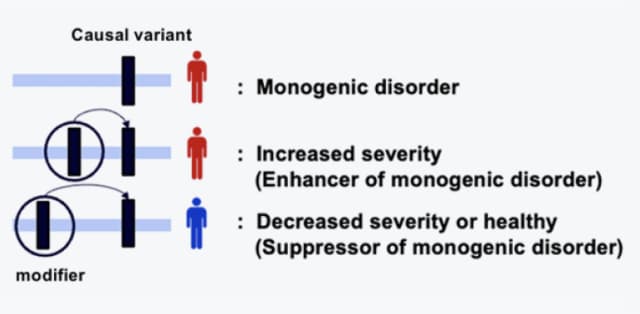
Pocket discovery
- Find effective pockets even without references based on the variant interpretation
- The active site indicates the site of the protein target which is both functional and pocket-like.
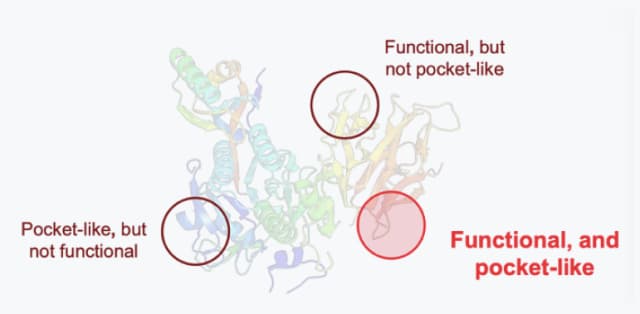
Active compound design
- Reaction based novel compound synthesis and active compound design methods
- Structurally novel but synthesizable compounds generated by AI
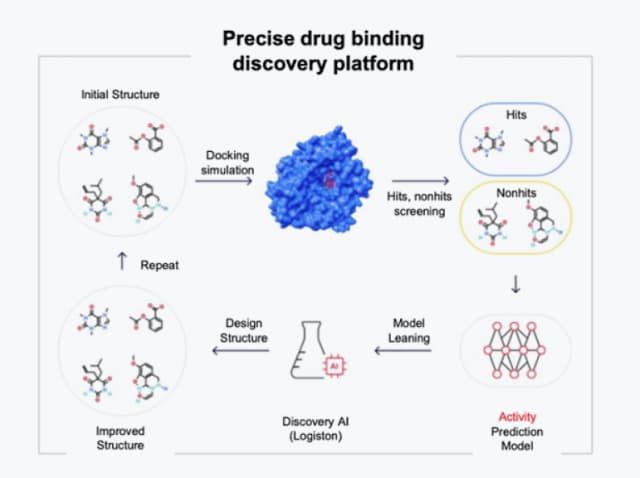
Synthesis simulation and discovery of new materials
MIN-T (Molecule Investing Network-Target binding) increases the probability of finding viable candidates through simulation of existing synthesis data and learning vast amounts of publibly available synthetic data. MIN-T was built by combining more than 100 synthesis methods with over 100,000 commercially available drug molecules.
Discovery of compounds with desired properties
MIN-T can design a compound to present desired physical properties, such as size, volume, reactivity, and solubility, through reinforcement learning. Compounds designed by MIN-T show a 50% higher accuracy in physical properties compared to compounds designed by AI-based compound generation models using well-known deep learning methodologies.
Optimized compound design for target binding
Existing methodologies are limited in that they can only find materials with high binding affinity from a large commercial library or create a similar substance by mimicking a known binding substance. In addition, out of the many different materials found in commercially available libraries, only a select few can actually be produced in a lab. Also, there are not enough materials that can be imitated due to information availability or patents. This presents a significant challenge to currently available compound design tools. MIN-T is able to create a variety of compounds optimized for target binding by combining different methodologies such as Docking, QSAR, and reinforcement learning. MIN-T reduces time and increases accuracy of drug discovery by applying Hit to Lead (H2L), a widely used drug candidate discovery method.
AI-Driven Drug Discovery
Accelerating Drug Discovery with AI technology: currently discovering lead compounds which are structurally novel and effective to the target designed by AI.
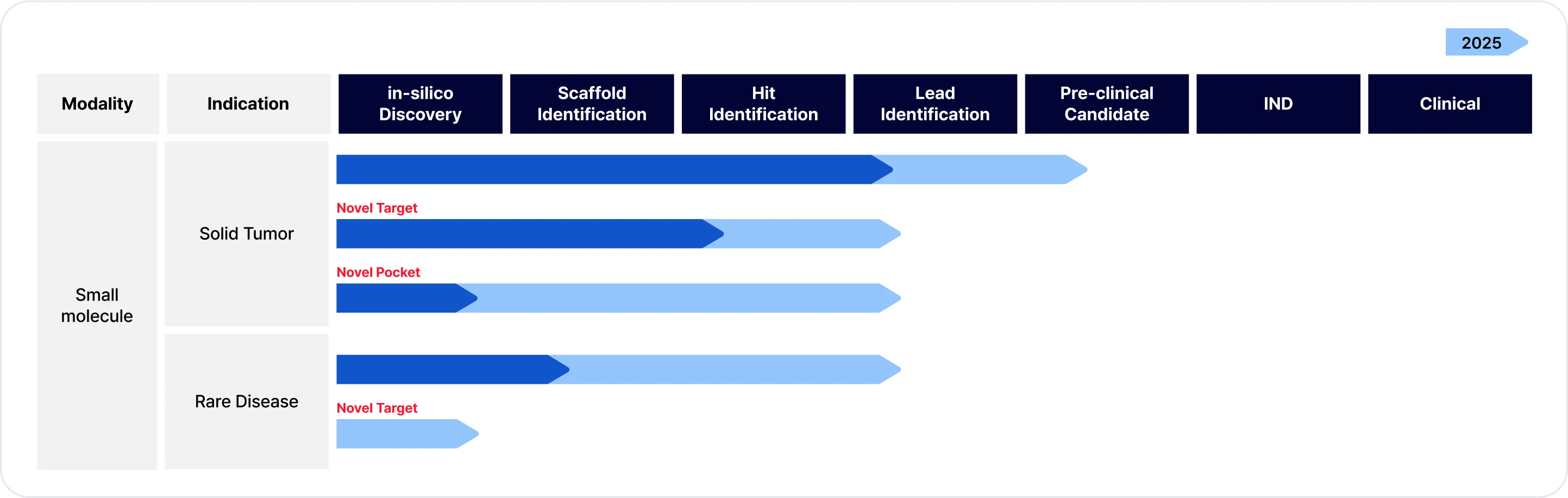
Initial tumor targets were selected to validate the AI system. Planning to expand rare diseases pipelines gradually from 2025.