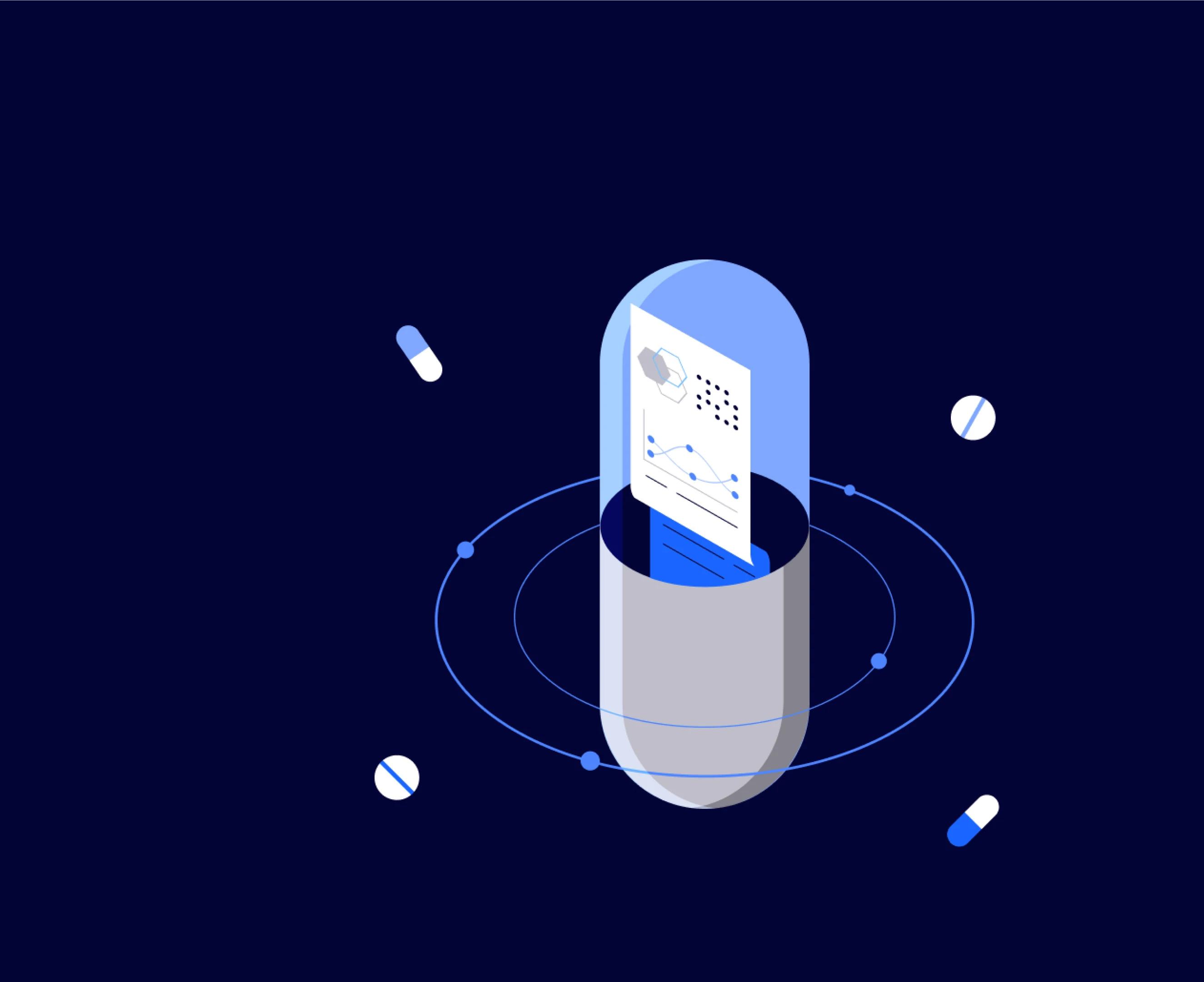
discovering new drug candidates
Limitations of existing deep learning drug discovery models
There have been attempts to create new compounds using deep learning methodologies.
However, there are limitations, such as finding a candidate with sufficient binding ability to the drug target, a candidate that can actually be produced in a lab, and a candidate that has enough flexibility to be optimized for many properties.
Application of AI systems to drug discovery

Synthesis simulation and discovery of new materials
MIN-T (Molecule Investing Network-Target binding) increases the probability of finding viable candidates through simulation of existing synthesis data and learning vast amounts of publibly available synthetic data. MIN-T was built by combining more than 100 synthesis methods with over 100,000 commercially available drug molecules.
Discovery of compounds with desired properties
MIN-T can design a compound to present desired physical properties, such as size, volume, reactivity, and solubility, through reinforcement learning. Compounds designed by MIN-T show a 50% higher accuracy in physical properties compared to compounds designed by AI-based compound generation models using well-known deep learning methodologies.
Optimized compound design for target binding
Existing methodologies are limited in that they can only find materials with high binding affinity from a large commercial library or create a similar substance by mimicking a known binding substance. In addition, out of the many different materials found in commercially available libraries, only a select few can actually be produced in a lab. Also, there are not enough materials that can be imitated due to information availability or patents. This presents a significant challenge to currently available compound design tools. MIN-T is able to create a variety of compounds optimized for target binding by combining different methodologies such as Docking, QSAR, and reinforcement learning. MIN-T reduces time and increases accuracy of drug discovery by applying Hit to Lead (H2L), a widely used drug candidate discovery method.