Orphan drug discovery using genomic data and artificial intelligence
- Insights | 22. 05. 12
According to the statistics, orphan drugs accounted for 58% of the newly approved drugs in 2020 and the total sale of orphan drugs accounted for approximately 20% of the pharmaceutical market in the United States.1 Orphan drug sales are growing at a much faster rate (11.3% growth rate) than overall drug sales (6.4%).2 Advancement in the personalized medicine industry appears to have accelerated this trend. As a result, orphan drugs along with targeted cancer drugs have emerged as key players in the recent novel drug development (Figure 1). However, pharmaceutical therapy is reportedly available for the treatment of only 5% of rare disorders.3 The development of first-in-class drugs would be extremely beneficial for many patients with rare disorders, who account for nearly 8% of the global population.3
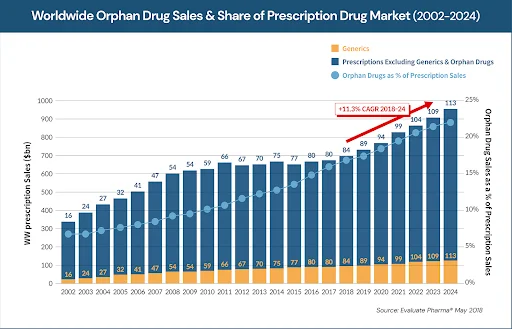
Figure 1. Emergence of orphan drugs in the pharmaceutical market
However, gene therapy, which is the primary treatment for genetic disorders, is relatively challenging to develop, resulting in extremely high drug prices and difficulty in finding health insurances that cover gene therapy4. Small molecule drugs can cover a broader range of rare disorders, and drugs available for oral administration could considerably improve the quality of life of patients. Furthermore, the cost of developing orphan drugs should be substantially reduced so that their price is comparable to that of drugs prescribed for common disorders. Many of the challenges associated with orphan drug development lie in candidate drug discovery and stem from the lack of information available about rare disorders, thereby making it difficult to identify effective targets and ligands.
What makes orphan drug discovery difficult
There are two major challenges associated with the discovery of orphan drugs. First, recruiting an adequate number of patients with different types of rare disorders is extremely difficult. The number of patients varies for rare disorders, and for several disorders, only a small number of patients are available as representatives of the population. Therefore, understanding the disorder and its mechanism of action becomes difficult. Although encountering a difficulty such as this appears to be inevitable in case of rare disorders, other rare disorders with similar symptoms and mechanisms should be studied to better understand each rare disorder.
Second, it is difficult to identify drug targets and ligands for these targets using traditional drug discovery methods. There is a lack of literature on rare disorders, and there are few biomarkers and experimental models available to assess the efficacy of drug candidates. Most disease and target databases and in-silico prediction tools are skewed to a large extent toward common disorders. The targets of rare disorders may differ substantially from those of common disorders. Nonetheless, previous chemical libraries were developed considering only common targets and disorders, which makes them ineffective for the identification of effective drug candidates for rare disorders. We believe that the use of genomic data and artificial intelligence (AI) can address many of these problems.
How genomic data helps discover orphan drugs
For orphan drug discovery, novel targets and effective therapeutic agents must be identified using relatively small amounts of information. We propose two solutions based on various types of genomic data.
(1) Selection of the target for each disorder using the overall genomic data on rare disorders
This strategy is based on the premise that “rare disorders are individually rare but cumulatively common.” Although our understanding of each disorder is limited owing to the shortcomings of previous research and literature, the rapid increase in genomic data, including whole-exome sequencing (WES) and whole-genome sequencing (WGS), has advanced our understanding of the relationship between gene and genetic disorders. Furthermore, using such data, protein targets that are translated from the genes could be better understood. Through the analysis of genomic data, the effect of functional changes in genes can be more specifically estimated. The effects of gene loss of function (LoF) and gain of function (GoF) are similar to those of target down-regulation and up-regulation.5 As a result, genomic data indicating the relationship between genotype and phenotype can assist in understanding the effects of target regulation. For some targets, both effects of gene LoF and GoF are known, which is referred to as a bidirectional effect. In comparison with drug targets with no known bidirectional effect, drug targets with known bidirectional effect have a 3–4-fold higher odds ratio of successfully passing clinical trials (figure 2).6
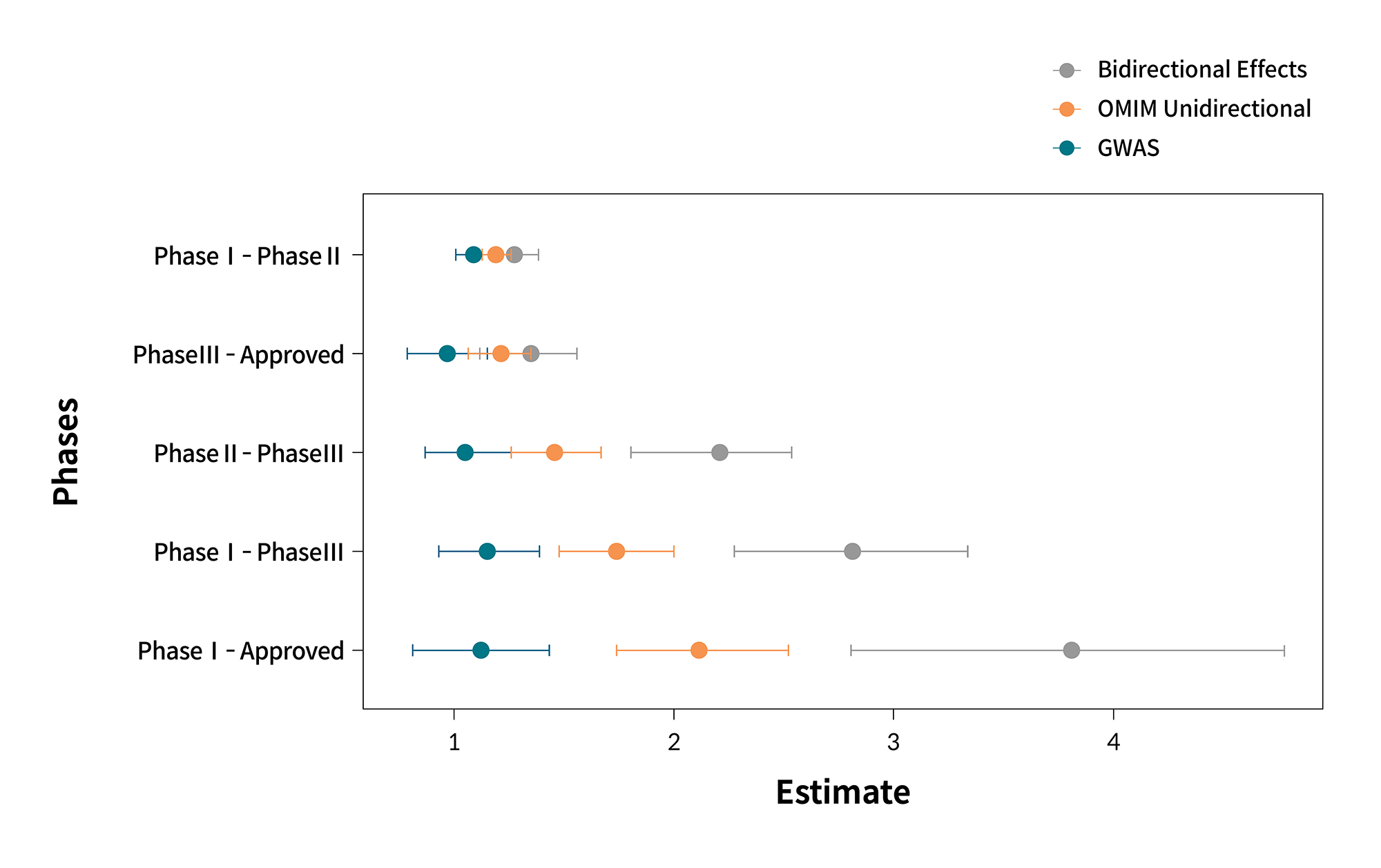
Figure 2. Generalizability of the bidirectional effect to increase the probability of success in clinical trials.
Such target effects estimated by genomic data are of particular significance in case of rare disorders. Genomic data from patients with rare disorders include the relationship between genetic variants and symptoms, which is extremely useful for target identification. There are different drug discovery cases based on genomic data from patients with rare disorders (Figure 3).7 Because the targets for rare disorders are rarely known by previous research, this approach is useful for their identification. It should be noted that causal genes do not have to be drug targets for genetic disorders. Other genes associated with the mechanism of action could be used as treatment targets, and information from other rare disorders could be used to identify the best target.
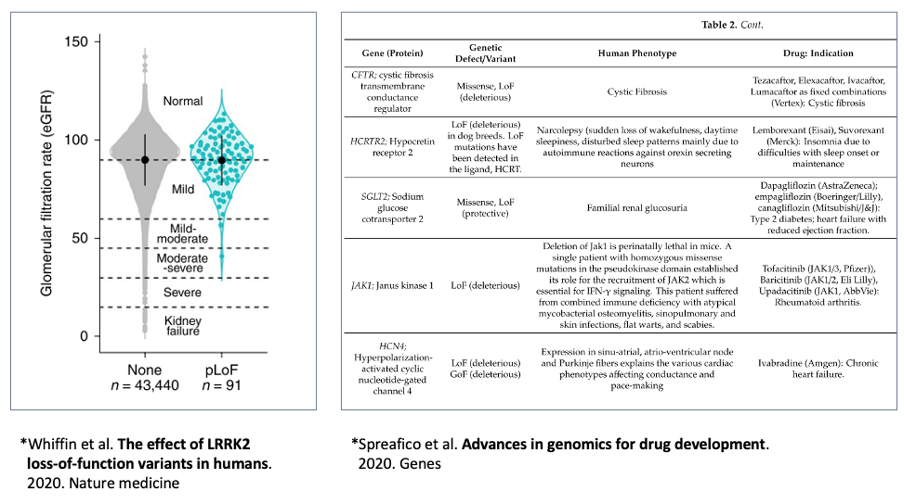
Figure 3. Cases that utilized genomic data to identify drug targets
(2) Finding active sites to control target activity
Effective drugs must bind to specific regions of their targets, referred to as “active sites” to alter their bioactivity. These active sites may be unknown in the case of novel targets for rare disorders. However, based on the genomic data on the functional changes caused by genetic variants, such active sites can be predicted (Figure 4). Such active site in the target can be predicted using the pathogenicity of the variants which occurred at that site. The variant sites with a higher probability of pathogenicity are more likely to be active sites of the target.8 Furthermore, by assessing the likelihood of variants altering the activity of the target, it would be possible to identify sites to downregulate or upregulate the targets more effectively.
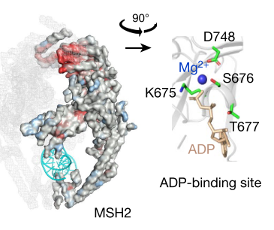
Figure 4. Identification of active sites by assessing the pathogenicity of variants on the target.
Although genomic data can be used to identify promising targets and active sites for drug discovery, a critical problem associated with such data is they are biased toward common disorders, genes, and variants. To overcome such biases and quantify the target effect, AI trained using genomic data should be employed. Furthermore, AI can be utilized to design chemical hits and leads to bind to the novel targets, thus altering their activity so that they can treat rare disorders.
Can AI accelerate orphan drug discovery?
3billion is attempting to use patient-centric genomic data gathered through genetic tests for patients with rare disorders to develop AI that could be useful in the discovery of orphan drugs. First of all, we are developing AI systems to evaluate target candidates based on the functional change in genetic variants (LoF and GoF). A disorder is defined as a combination of symptoms, and the genes associated with such symptoms could be treatment targets. For instance, a symptom caused by a gene GoF can be treated by downregulating the gene target. As a result, an AI trained in identifying the functional changes caused by genetic variants can assess the suitability of targets for treating specific symptoms or disorders. Assessed scores can be used to prioritize targets, allowing the most promising targets to be thoroughly examined. Furthermore, the AI-based text-mining system that we developed can assist in the analysis by increasing the efficiency of literature mining.
Furthermore, 3billion is developing generative AI to design drug candidates that can bind to the designated target and active site. As stated previously, based on the information regarding variant pathogenicity, potential active sites can be identified. After determining the target and active site, molecular ligands likely to bind to the active site can be designed by employing molecular simulation based on the structures of molecules (Figure 5). We developed a drug designing AI that can generate ligand structures, which can be synthesized using known chemical reactions and have drug-like molecular properties. It can also help design molecules having desirable pharmacokinetics based on the target indication for drug discovery. Thanks to generative AI, we can explore diverse ligand structures that have not been designed before, thereby avoiding the bias that conventional chemical libraries have.
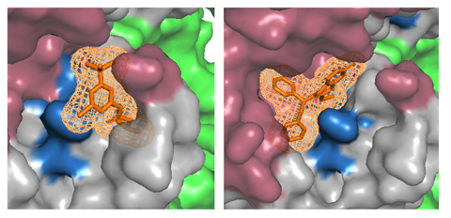
Figure 5. Designing effective chemical ligands that can bind to the target active site.
Conclusion
Several studies have demonstrated that the most effective target for a specific disorder or symptom can be identified by analyzing the genomic data of the patients. Because there is a dearth of prior research and literature on rare disorders, this approach is advantageous. However, because of the biased and unstructured nature of genomic data, only few studies have been conducted to identify drug targets using genomic data quantitatively. 3billion is gathering patient-centric genomic data through genetic testing, such as WES and WGS, and applying well-structured analytic tools to gain important information regarding the relationship between genetic variants and symptoms. These data can be trained by AI and used to identify the most effective target and active site, as well as hits and leads for the treatment of a particular disorder. As a result, we hope that AI technology will substantially reduce the time and cost required for orphan drug discovery, while also increasing the likelihood of successful investigational new drug approval. Such technological progress would accelerate orphan drug development, allowing for the treatment of various disorders and symptoms, in addition to an improvement in the quality of life of patients with rare disorders.
References
- NEXT Report. A time for resilience and ingenuity. 2021. Global genes.
- The search for Treatments and Cures. 2019. Global genes.
- Chris Austin. RARE Drug Development Symposium. 2020. Global genes.
- Drugs.com. Why is Zolgensma so expensive?
- Whiffin et al. The effect of LRRK2, loss-of-function variants in humans. 2020. Nature medicine.
- Estrada et al. Identifying therapeutic drug targets using bidirectional effect genes. 2021. Nature Communications.
- Spreafico et al. Advances in genomics for drug development. 2020. Genes.
- Frazer et al. Disease variant prediction with deep generative models of evolutionary data. 2021. Nature.
Do you find this post helpful?
Click the button below to copy and share the link.
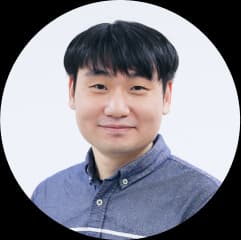
Kyoungyeul Lee
AI engineer specializing in genome analysis and drug discovery, leading AI-driven variant interpretation and drug development for rare diseases at 3billion.